Chromatography plays a vital role in separating and analyzing complex mixtures. Its broad applications provide crucial insights for quality control and research and development, making it an essential technique in industrial R&D, from pharmaceuticals to environmental science. However, several factors, including the use of resources (consumables and solvents), waste generation, disposal of solvents, and energy consumption, contribute to its significant environmental impact.
Continual efforts are being made to reduce the environmental footprint of chromatographic processes. While some waste is inevitable and technical challenges, regulatory issues, economic constraints, scalability, and other difficulties emerge in creating greener methods, it is still possible to improve sustainability. The adaptation and application of the 12 Principles of Green Chemistry formulated by Paul Anastas and John Warner1 to chromatographic processes and workflows help to minimize environmental impact without sacrificing efficiency and accuracy.
Approaching Sustainability in Analytical Labs: Adaptation of Green Chemistry Principles
Organizations are adopting green chemistry principles to transform existing chromatography practices and reduce their environmental impact. To make analytical labs more sustainable, it is necessary to implement strategies such as reducing and replacing solvents, designing workflows to improve energy efficiency, and conducting in-silico experiments to minimize waste.
Reduce Solvents and/or Replace with Safer Solvents and Auxiliaries
Solvents are extensively used in chromatographic processes, and their environmental impact can be attributed to their source/synthesis, properties, and disposal. Green chemistry principles dictate the importance of selecting the most suitable solvent to minimize environmental impact. To aid in selecting greener solvents, the pharmaceutical industry has developed solvent selection guides, such as the ACC GCI-PR guide.2 However, it is important to note that a greener solvent does not always equate to a more sustainable process—multiple factors must be considered.
A key strategy for greener chromatography is reducing the volume of solvents used. While solvent-free methods would be ideal, they often lack the necessary selectivity and sensitivity. In such cases, replacing harmful solvents with greener alternatives such as supercritical fluids or ionic liquids, can significantly reduce environmental impact.
Design for Energy Efficiency
Energy is consumed through chemical and pharmaceutical processes, contributing significantly to their environmental footprint. The 12 Principles of Green Chemistry emphasize the importance of minimizing energy requirements.
Solvent removal is a major contributor to energy consumption, highlighting the need for careful solvent selection. Moreover, using energy-efficient instruments such as ultrahigh-performance liquid chromatography (UHPLC) enhances separation efficiency and shortens overall run time, reducing energy use significantly.
Maintaining the required temperatures and pressures for these processes also adds to their environmental impact. Conducting experiments at ambient temperatures and pressures and optimizing heating and cooling systems are essential to lower energy consumption.
Prevent Waste
According to green chemistry principles, preventing waste is preferable to treating/cleaning it once it has been created. Greener chromatography can be achieved by developing methods that require smaller sample sizes and generate less waste. The use of predictive software tools to develop and optimize these methods in silico helps minimize trial-and-error method development and unnecessary experimentation, further preventing waste generation.
Sustainable Chromatography using Software Tools
Waste is inevitable when conducting chemical experiments. When it comes to chromatography, the most eco-friendly experiments are those performed on a computer. The right software solutions can improve the sustainability of chromatographic experiments, significantly designing and optimizing methods that align with green chemistry principles. Software with predictive tools and method simulation enables optimization with fewer experiments—promoting greener practices and facilitating smarter decision-making.
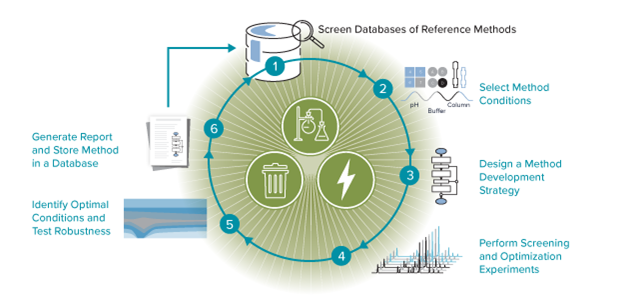
Avoid Unnecessary Experiments with Predictive Technology
A deep understanding of the physicochemical properties and likely behavior of a molecule, along with the ability to rationally select chromatographic parameters, are required to develop, refine, and improve methods with minimal trial-and-error experimentation. A good foundation for this understanding comes from the expertise and knowledge of scientists. However, there are limitations to this approach, such as cognitive capacity, potential biases, and manual analysis (often a slow process), which must be overcome. Building on this with a computational approach leads to more robust and insightful scientific outcomes.
Software prediction tools that use quantitative structure-property relationship (QSPR) calculations and complex algorithms to predict physicochemical properties such as logP, logD, pKa, and more are of immense value. Predictions generated with software tools offer high accuracy, consistency, speed, scalability, and the ability to manage substantial amounts of complex data.
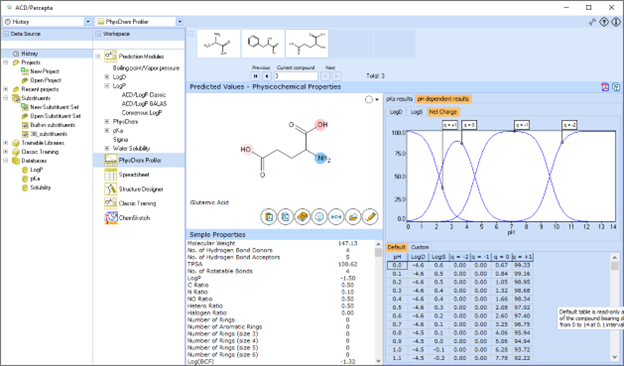
Additionally, method development software tools such as column selection, column comparison, and pH selection can help predict a better starting point for method development. Combining these tools with a systematic and rational approach can help to quickly identify and select optimal chromatographic parameters while reducing the number of experiments required.
Combining scientists’ expertise and leveraging intelligent software tools ensures reproducible and comprehensive scientific analysis while conserving resources by minimizing the number of experiments.
In Silico Modeling—Optimize Chromatographic Simulations, Reduce Waste, and Save Time
In silico modeling allows scientists to identify the most promising experimental conditions while minimizing unnecessary physical experiments. Using in silico modeling offers scientists increased flexibility in testing different scenarios and adaptability to easily adjust and re-run models to accommodate new data or changing experimental goals.
Early identification of optimal conditions and rapid simulations via in silico modeling accelerates the method development process. Complex algorithms and extensive datasets used by method development software help scientists quickly predict the most optimal chromatographic conditions (pH, gradient, etc.). They are also able to better separate peaks using 1D, 2D, or 3D models. Mathematical parameters in these software tools help scientists quickly select parameters that best meet their defined success criteria (k,’ run time, resolution, and peak relevance). Software features such as multivariable analysis and visualization of data help scientists better understand and interpret complex datasets.
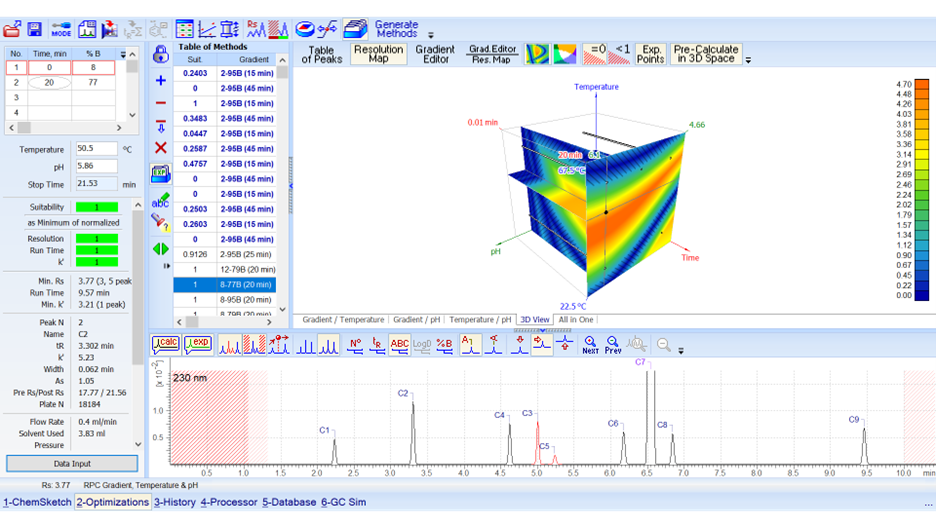
Improve Sustainability with Enhanced Data Management
Labs usually operate with a variety of instruments from different vendors, leading to copious amounts of unstructured and isolated data. This can pose several challenges, such as higher susceptibility to errors and loss of data, which often means that experiments are duplicated. To achieve more sustainable research and development, it is essential to have efficient and effective data integration and management.
This can be achieved with vendor-neutral and platform-agnostic tools that provide a solution to capture, process, and analyze multiple file formats and data types into a single standardized data format within a single interface. Having standardized files and formats makes data easier to process and analyze, reducing the time scientists spend assembling and processing data.
The ultimate solution combines data standardization with data management systems to store and organize live analytical and chemical information (methods, structures, metadata, etc.) within centralized, searchable databases. These databases connect data to original experiments for simplified review and verification and give scientists easy access to all pertinent information to help them make informed data-driven decisions. Collaborative work environments are further facilitated, allowing scientists and organizations to work together seamlessly with software features that generate detailed customizable reports. Enhanced data management ensures that knowledge is preserved and leveraged for reproducible research.
Improving Sustainability with Artificial Intelligence (AI)
AI contributes to sustainability in analytical labs through the optimization of processes using real-time monitoring and adjustment, predictive analytics for sustainable experiment design, enhanced data management, and automation of routine tasks to reduce redundant work.
The quality of data is crucial for unlocking the full potential of AI. High-quality datasets must be standardized, comprehensive, and prepped for use with advanced analytical tools (such as data science, AI, or machine learning). As datasets increase in number, size, and diversity, the demand for efficient and effective data analysis increases. Having data that is ready for advanced analytical tools is necessary to facilitate data analysis, create predictive models, and accelerate the prediction and optimization of in silico experiments.
Advancing Sustainability in Analytical Labs
With the growing focus on sustainability, adopting green chemistry principles is becoming increasingly important. The environmental impact of chromatography, a vital analytical tool, can be significantly reduced by embracing green practices. Although challenging, sustainable methods can be implemented without compromising efficiency or accuracy. Enhanced software tools, including predictive technology, in silico modeling, and data standardization, not only align with green chromatography principles but enable scientists to perform fewer experiments, use resources responsibly, and manage data effectively. Incorporating these tools into laboratory workflows ensures that data is ready and accessible by scientists and AI and ML applications. With access to comprehensive and historical data, scientists can better manage their data and draw deeper insights, all while saving time and money. Embracing green chromatography enables scientists to maintain high-quality results and contribute to a more sustainable future.
Meet the Expert
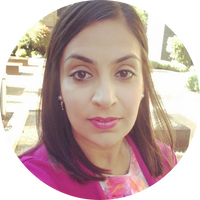
Baljit Bains
Baljit Bains is a Marketing Communications Specialist at ACD/Labs. Her main focus is on analysis of mass spectrometry and chromatography data. Prior to joining ACD/Labs, Baljit earned her Master of Pharmacy at Aston University and her Honours Bachelor of Science at the University of Toronto.
References
- Anastas, P. T.; Warner, J. C. (1998). Green Chemistry: Theory and Practice. Oxford University Press.
- ACS Green Chemistry Institute® Pharmaceutical Roundtable. Solvent Selection Guide: Version 2.0, March 21, 2011. Retrieved on November 8th, 2024 from https://www.acs.org/greenchemistry/research-innovation/tools-for-green-chemistry.html
This article is featured in our March 2025 publication, Redefining Analytical Boundaries. Explore this issue to unlock AI-driven efficiency, advanced mass spectrometry insights, and sustainable chromatography breakthroughs.
